Major Challenges with AI Compute Networks & How DePINs Can Solve It
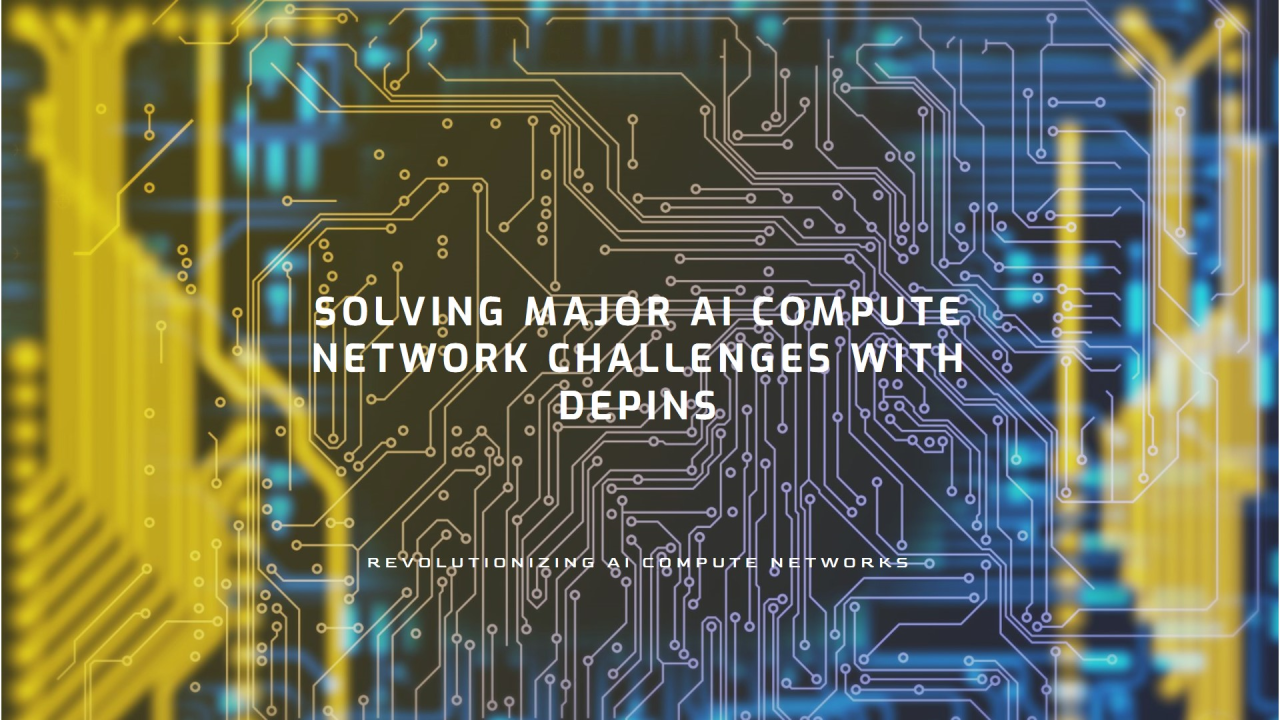
Sam Altman’s ambitious goal to become independent from NVIDIA by seeking $7T investment raged the internet just a few weeks leading up to NVIDIA’s quarterly income announcement which skyrocketed by 768.81% for Q1, 2024. These are perfect examples of the obvious - AI’s demand for hardware is here to stay and is likely to reshuffle the power play between the tech giants.
The major challenge with AI compute networks involves several interconnected issues, primarily revolving around scalability, efficiency, and accessibility:
- Scalability: As AI models become increasingly complex, the computational resources required to train and run these models grow exponentially. This raises challenges in scaling compute infrastructure to meet these demands without incurring prohibitive costs or environmental impacts.
- Accessibility and Centralization: The high costs and technical expertise required to build and maintain AI compute networks lead to centralization, where only a few large organizations can afford to engage in cutting-edge AI research. This centralization raises concerns about accessibility for smaller entities and individuals, potentially stifling innovation and diversity in AI development.
- Resource Allocation and Management: Efficiently managing the available computational resources, including hardware (CPUs, GPUs, TPUs) and software (optimization algorithms), to reduce bottlenecks and maximize throughput is a complex challenge. This also includes the development of better tools and frameworks to streamline AI workflows.
- Data Management and Storage: The large datasets required for training sophisticated AI models necessitate extensive storage solutions and efficient data management practices to ensure that compute resources are not wasted on data handling inefficiencies.
- Energy Efficiency and Environmental Impact: The massive energy consumption of large-scale AI computations has significant environmental impacts, including high carbon footprints. Improving the energy efficiency of AI compute networks and finding sustainable energy sources for these operations are critical challenges.
There is a better way. NeurochainAI is connecting community-owned GPUs and CPUs into a Decentralized Physical Infrastructure Network (DePIN) to address the key challenges mentioned above. NeurochainAI’s DePIN is infinitely scalable through the network of decentralized consumer-grade hardware that doesn’t require increased energy resources compared to some of the new GPUs built specifically for AI. On top, tapping into community-owned resources increases sustainability by using what’s already out there instead of building new machines. This also enables the community to play a role in the AI revolution which so far has been limited to OpenAIs, Googles, and Metas of the world.
Of course, the new decentralized AI compute networks are not without their own limitations which include limitations on what type of AI models can run based on the GPUs in the network or data handling in a decentralized network. These are important topics that the AI developer community as a whole is addressing as we speak. However, the most important aspect is that the AI compute DePINs present an alternative to the centralized “black-boxed” AI future that only a few biggest corporations control.